As
mentioned earlier, epidemiologists used several different types of
studies. Simply speaking, these can be classified as either experimental,
where the epidemiologists have control over the circumstances from the
start, or observational, where they do not. Vaccine efficacy trials
are a good example of experimental studies because investigators control
who gets the vaccine and who doesn't. Observational studies can be further
subdivided into descriptive and analytical studies. In a
descriptive study, the epidemiologist collects information to characterize
and summarize the health event or problem. In an analytical study, the
epidemiologist relies on comparisons between groups to determine the role
of various risk factors in causing the problem. Descriptive epidemiology
is the most basic of the these categories and is fundamental to the work
of an epidemiologists.
Another way
of comparing descriptive and analytical epidemiology is to say that in the
descriptive process, we are concerned with "person" (Who was
affected?), "place" (Where were they affected?), and time (When
were they affected?). Once we know the answers to these questions, we can
enter the realm of analytical epidemiology and ask how and why these
people were affected.
A
descriptive study of fatalities associated with the use of farm tractors
illustrates the usefulness of person, place, and time for drawing
inferences about health problems. The study was conducted in the early
1980s, using data from death certificate records, which are a readily
available surveillance system. Take a moment to study the graphics below
and consider what the data might mean, then click the
"Inferences" bar for an explanation.
Back to Top
Deaths
associated with tractor injuries, by month of death, Georgia 1971-1981
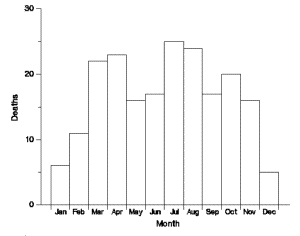
Deaths peaked in the spring and fall
months, so they may be related to planting and harvest.
Deaths
associated with tractor injuries, by time of day, Georgia 1971-1981
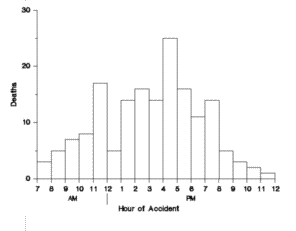
Because deaths peaked just before lunch
and during the late afternoon and decrease from 12:00-1:00, they might be
related to fatigue. The dip at noon could be because most people are
taking a break for lunch. The fact that children are home from school by
4:00 could contribute to the peak in the afternoon.
Back to Top
Deaths associated with tractor injuries, by place, Georgia
1971-1981
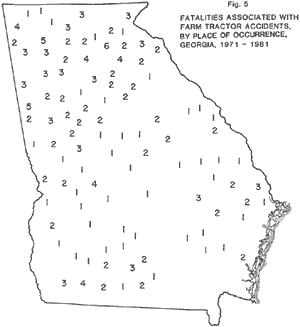
Most of the deaths occurred in northern
Georgia, which is hilly and mountainous; south-central Georgia, where
fewer deaths occurred, is much flatter. This distribution might implicate
the rugged terrain, but because the map shows numbers of deaths, not
rates, we don't know whether the numbers could instead reflect differences
in population size or even perhaps the number of tractors being used. As
for the prevalence of tractors, south-central Georgia is more agrarian
than northern Georgia, so the number of tractors probably isn't a factor.
Another possible association is differences in experience and skill in
using tractors.
Back to Top
Deaths
associated with tractor injuries, by age group, Georgia 1971-1981
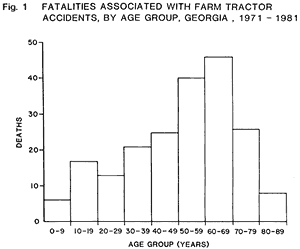
The number of deaths is clearly higher in
the older age group. This could mean that tractor users are predominantly
older, but it could also indicate that older users are less likely to
survive an accident.
Any inferences you make are likely bases
for hypotheses, which would then have to be tested using one of three
analytical study designs: cross-sectional, cohort, and case-control. In
all three types, the epidemiologist is attempting to discover the
relationship between an exposure or risk factor and a health outcome. For
example: Did the chicken salad at the company picnic cause the salmonella
outbreak? Does cigarette smoke cause lung cancer? Are alcohol use and
motor vehicle crashes related? Does the supplement L-tryptophan cause EMS?
The first type of design, a cross-sectional
study, is basically the same as a survey. In this type of study,
the epidemiologist defines the population to be studied and then collects
information from members of the group about their disease and exposure
status. Since the data represent a point in time, it's like taking a
"snapshot" of the population. Cross-sectional studies are good
for examining the relationship between a variable and a disease, but not
for determining cause and effect, which requires data over time. Cohort
studies and case-control studies are much better suited to examining
cause-and-effect relationships.
In a cohort study, you
select the study population according to their exposure, regardless of
whether they have the disease or health outcome you're studying. You then
determine the outcomes and compare them on the basis of the individuals'
exposures. Cohort studies are often referred to as prospective
studies because they follow the study population forward in time, from
suspected cause to effect. An example would be dividing a group of people
on the basis of their smoking status and following them for 20 years to
see if they develop lung cancer. Cohort studies are also used to
investigate outbreaks in small, well-defined populations. For example, you
would use a cohort study to answer the question posed earlier regarding
the cause of a salmonella outbreak at a company picnic. In this situation,
you would ask each attendee about their exposure (e.g., what foods and
beverages they consumed at the picnic) and whether they became ill
afterward. The relationship between exposure and outcome in a cohort study
is quantified by calculating the relative risk for the
exposure.
Back to Top
Cohort studies have several advantages:
- You can examine multiple outcomes for
a single exposure. For instance, if you select a group based on their
smoking exposure, you can look not only at the incidence of lung
cancer, but also at the incidence of cardiovascular disease,
emphysema, burns, other smoking-related outcomes.
- Cohort studies are very useful in
examining rare exposures, such as asbestos exposure and lung cancer.
- You can directly calculate the
incidence of disease for each of the exposure groups.
- The temporal sequence is logical: you
are starting with exposure and following forward in time to the
development of disease.
Disadvantages of cohort studies are that
they are costly in time and resources and, if the disease is rare, you
need a large number of subjects. Also, because you are following forward
in time, logistical problems may develop and subjects can be lost to
follow-up. (Cohort studies are discussed in more detail in
How
to Investigate an Outbreak.)
In a case-control study,
the epidemiologist is working backward, from the effect to the suspected
cause. For this reason, case-control studies are often referred to as retrospective
studies. Participants are selected on the basis of the presence or absence
of the disease or outcome in question, so that you have one group of
people (case-subjects) with the health problem and one without (controls).
These groups are then compared to determine the presence of specific
exposures or risk factors. For example, you could pick a group of people
with lung cancer and a group without and then compare them for their
history of exposure to smoking. The relationship between exposure and
outcome in a case-control study is quantified by calculating the odds
ratio.
Back to Top
Case-control studies have three primary
advantages:
- You can examine multiple exposures for
a single outcome.
- They are well suited for studying rare
diseases and those with long latency periods.
- They require fewer case-subjects and
are generally quicker and less expensive to conduct than cohort
studies, which makes them well suited for the conditions of an
outbreak investigation.
The disadvantages of case-control studies
are that they aren't suitable for studying rare exposures;
they are subject to bias because of the
method used to select controls; and they don't allow you to directly
measure the incidence of disease. Also, because they look backward,
case-control studies may create uncertainty about the temporal
relationship between exposure and disease. (Case-control studies are
discussed in more detail in How to Investigate an
Outbreak.)
One important point must be made about
analytical epidemiologic studies: In these studies, we are attempting to
answer the how and why questions, and we are able to quantify the
relationship between an exposure and an outcome. However, a mathematical,
or quantitative, relationship between the two is not enough to establish
causation. We can demonstrate increased relative risk of lung cancer for
smokers in a cohort study, or we can demonstrate in a case-control study
that people with lung cancer are much more likely to have smoked in the
past; but that alone doesn't establish cause and effect.
In general, five criteria must be met to
establish a cause-and-effect relationship:
- Strength of associationthe
relationship must be clear.
- Consistencyobservation of the
association must be repeatable in different populations at different
times.
- Temporalitythe cause must precede
the effect.
- Plausibilitythe explanation must
make sense biologically.
- Biological gradientthere must be a
dose-response relationship.
Back to Top | Next Topic:
Disease Transmission
|